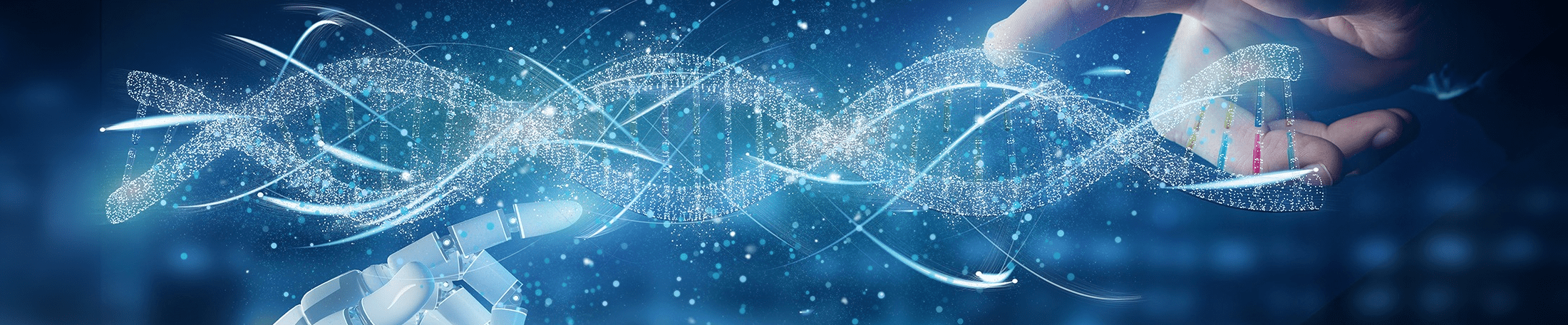
Second round of WASP and DDLS joint research projects
During 2022/23, WASP and the SciLifeLab and Wallenberg National Program on Data-Driven Life Science (DDLS), the two largest research programs in Sweden, launched a second joint call with the aim of solving ground-breaking research questions across their different scientific disciplines. In total, 13 applications were awarded grants for two-year projects.
The call closed on May 24, 2023, but you can read about the call here.
Researchers: Nina Linder (UU), Claes Lundström (LiU)
Background: Cervical cancer is the fourth most common cancer among women worldwide, and about 90% of the new cases and deaths occur in low- and middle-income countries. Linder et al have previously developed an AI-based point-of-care (POC) diagnostic system for cervical cancer screening in resource-limited settings. However, domain shift poses challenges for large-scale implementation of AI-based diagnostic methods, as AI systems may yield unreliable predictions when encountering data that differs from the training data.
Aim: To develop computational methods based on uncertainty estimation, to tackle domain shift challenges in AI applications for digital cytology and to integrate the methods into a workflow in a prospective study for improved human-and-machine interplay in the diagnostic process.
Methods: The project will explore methods to mitigate the domain shift challenges, focusing on uncertainty estimation to increase prediction robustness or detect mispredictions. A prototype clinical viewer, including uncertainty estimations will be evaluated within a prospective clinical study. The assumption is that uncertainty predictions will allow human experts to focus on challenging cases, while high-confidence AI predictions require minimal human intervention.
Significance: The project has significant societal and industrial impact potential by enabling more accurate, efficient, and accessible diagnostics for cervical cancer, also in resource-limited settings.
Researchers: Volker Lauschke (KI), Ming Xiao (KTH)
Background: Chemotherapy is essential in the treatment of several cancers; however, drug resistance remains a substantial problem. To predict chemotherapy response, certain genetic variations in the drug transporter genes MDR1, MRP1 and BCRP are currently used as clinically established biomarkers. In a recent study, genomic data from >130,000 individuals were analyzed, revealing more than 3000 novel genetic variations in these transporters with unclear functional consequences.
Aim: Develop a machine learning based tool for pharmacogenetic predictions of chemotherapy response in cancer treatment.
Methods: A substrate-specific functionality profile of all genetic variants of human drug transporters will be generated using deep mutational scanning followed by phenotypic selection in cancer cells. The resulting data set will be used for the development of graph neural network (GNN)-based learning schemes for pharmacogenetic predictions of drug response.
Significance: This project will facilitate the translation of an individual’s genomic information into chemotherapeutic sensitivity profiles, providing an important mechanistic link between rare genetic variations, transporter function and clinical outcomes. The long-term goal is to allow for more individualized therapy strategies to increase patient survival, but also to decrease the risk of severe side-effects treatments.
Researchers: Pelin Sahlén (KTH), Wojciech Chacholski (KTH)
Background: A deeper knowledge of human genetic variation and its complex interplay within genomic elements constitutes a cornerstone in the understanding of several human diseases. The 3D arrangement of the human genome reveals functional information by physically positioning regions that work in close proximity. Novel methods that can look at the 3D arrangement and covariation profiles of multiple locations in genome are highly needed.
Aim: develop a method to improve the understanding of sequence covariation and its connection to pathology.
Methods: sequence and functional datasets will be used to establish criteria for identifying when genetic elements, like genes, lincRNAs, promoters, or enhancers, either tend to appear together or exhibit differences in their patterns of variation. Topological Data Analysis (TDA) will be used to explore and the relative geometry of genomic topologies to find places that function together.
Significance: The long term goal is to go beyond linear analyses of the genome data by exploiting the genome folding constraints to obtain a glimpse of coordinated regulation of the genome and its impact in disease onset and progress.
Researchers: Fredrik Levander (LU), Lukas Käll (KTH)
Background: Molecular biomarkers are a prerequisite in contemporary precision medicine. Proteins are the functional entities in cellular processes; therefore, proteomics provides excellent possibilities to discover novel biomarkers. Proteoforms are different molecular forms or variants of a protein that can arise due to various post-translational modifications, alternative splicing of mRNA, or other molecular events, each of which may have distinct biological functions or properties. So far, proteoforms are to a large degree ignored in current proteomic studies, mostly due to shortcomings of current informatics workflows that fail to handle the complexity of data.
Aim: enable the differential quantification of proteoforms in sample cohorts, by leveraging the millions of mass spectra deposited in repositories using novel feature extraction and machine learning approaches.
Methods: spectral clustering, feature extraction and deep learning will be used to develop methods to detect modified proteins in deposited data. A machine learning framework to detect proteoform level differences within sample will be developed. The resulting algorithms will be used to reanalyze medically relevant datasets to detect novel biomarkers.
Significance: The project aims to transition proteomics from a gene-centric to a proteoform-centric perspective, thereby amplifying the opportunities to discover pertinent biomarkers.
Researchers: Björn Önfelt (KTH), Mårten Björkman (KTH)
Background: Natural killer (NK) cells are a vital part of the innate immune system through their capacity to detect and eliminate virus infected or transformed cells without prior activation. Cellular immunotherapy utilizes the immune system’s ability to recognize and kill malignant cells. For effective therapy it is important to harvest and expand immune cells that show effective anti-tumor responses. Current methods are mainly relying on isolating cells based on protein phenotype, often leading to cell populations that are very heterogeneous in terms of functional responses.
Aim: develop new, improved methods to automate the process of identifying NK cells with extraordinary ability to kill tumor cells.
Methods: Machine learning (ML) algorithms will be trained to distinguish NK cells displaying different levels of cytotoxicity using image data from single cell screens. By increasing content in the imaging data used for training, and include time-lapse sequences revealing cell dynamics, NK cell identification will be improved. A framework for fully automated AI-driven identification and harvest of NK cells with extraordinary cytotoxic potential will be forged.
Significance: Development of a framework for fully automated AI-driven identification and harvest of NK cells with extraordinary cytotoxic potential is assumed to significantly improve the efficiency of adoptive cell therapy in cancer treatment.
Researchers: Ville Kaila (SU), Simon Olsson (Chalmers)
Background: Mitochondria are membrane-bound cell organelles generating energy supplies in the form of ATP through oxidative phosphorylation. Complex I is the largest component of the mitochondrial oxidative phosphorylation system, and its dysfunction is linked to nearly half of all known mitochondrial disorders, with point mutation resulting in e.g. cancer and neurodegenerative diseases. Despite the significant structural and biochemical data over the last decades, their mechanistic principles remain poorly understood and a major challenge for life-sciences.
Aim: develop methods to quantitatively predict the biological reactivity and functional dynamics of the membrane proteins responsible for energy transduction in mitochondria, with focus on complex I.
Methods: Physics-based ML models will be derived using neural networks by combining highly accurate, but computationally challenging quantum simulations with biophysical experiments, evolutionary and mutagenesis data. The models aim to predict how the protein structure determines the biochemical activity and apply these to large-scale in silico-screening for probing how mitochondrial disease-related mutations alter the protein function.
Significance: The project will combine ML approaches with biochemical, biophysical, and structural data, providing a basis for understanding how proteins power the energy metabolism of our cells, the evolution that led to the emergence of these intricate biological complexes, and how human disease related mutations alter the protein function.
Researchers: Anna Rising (SLU), Hedvig Kjellström (KTH)
Background: Due to its impressive strength, material properties, and sustainability, artificial spider silk is a highly desirable material that could potentially be used in a vast number of applications. However, artificial replication of spider silk has turned out to be much harder than previously thought and artificial fibers are inferior to native silk. Recently, a new study revealed the transcriptomes from more than 1000 silk glands and corresponding data on silk fiber mechanics that might help solve this mystery.
Aim: To use machine learning on the available data to reveal the unique factors that give native spider silk its strength, and to test the results with previously developed in-house methods.
Methods: By utilizing machine learning on the data from the new study, such as expression levels, protein molecular weight, hydrophobicity, secondary structure (predicted by AlphaFold2), and amino acid residue composition, the project PIs plan to reveal the unique factors that give native spider silk its mechanical properties. The researchers will then use their own unique biomimetic method for production of artificial spider silk fibers to verify the output from the artificial intelligence, and to spin fibers with mechanical properties that match those of native fibers.
Significance: If the project is successful, the results could have a major impact on the material science field and play an important role in the sustainability transition by providing materials with low negative impact on the environment. They could also facilitate the development of other proteinaceous high-performance materials.
Researchers: Malin Malmsjö (LU), Victor Olariu Ahnell (LU)
Background: Conventional diagnosis of skin cancer involves biopsy excisions followed by histopathological analysis. This invasive process, which can be painful for patients and often results in the removal of a larger area of skin than necessary, requires trained specialists and can take several days or even weeks to complete. Hyperspectral imaging, a relatively new technique that enables the analysis of a much wider spectrum of light than what our eyes can see, and photoacoustic imaging, a non-invasive technique that converts ultrasonic wave patterns generated by laser-heated tissue into detailed spatial images, might be the key to faster, more accurate, and non-invasive diagnoses.
Aim: To develop a machine learning model that can identify tumor borders orders of magnitude faster than the current state of the art methods, by analyzing hyperspectral and photoacoustic images.
Methods: Hyperspectral imaging will be used in combination with photoacoustic imaging to be able to analyze both the spectral molecular fingerprint and depth of skin lesions. Artificial intelligence will then be trained to accurately predict the correct diagnosis.
Significance: By using neural network models on high contrast images containing unique molecular information from hyperspectral and photoacoustic images, it is possible to streamline skin tumor diagnosis, making it both safer and faster for the patients. This will also reduce the medical costs as well as lower patient suffering by reducing the number of misdiagnosis and unnecessary re-surgeries.
Researchers: Henrik Hult (KTH), Peder Olofsson (KI)
Background: Bioelectronic medicine, an emerging discipline combining neuroscience, immunology, and electrical engineering, might lead to the development of new methods capable of monitoring and treating diseases by electrical intervention in the peripheral nervous system.
Aim: To create data-driven statistical and machine learning algorithms that can analyze electrical signals from the peripheral nervous system to predict the level of glucose in the blood, and to provide proof-of-principle that an autonomous machine can replace the sensory detection by the central nervous system of bodily functions, with potential applications that will range far beyond predicting glucose levels.
Methods: The data will be collected through an implanted electrode on the vagus nerve while blood glucose levels will be varied. Using a conventional device to measure blood glucose as a reference, training data from the electrode is generated as high-frequency multi-channel signals that can be analyzed using a combination of statistical signal processing techniques and machine learning algorithms.
Significance: By utilizing artificial intelligence and machine learning to interpret the recorded nerve signals from the peripheral nervous system, autonomous adaptation of treatment could potentially be achieved. This will help tackle the well-known dosage and timing problems, which are responsible for many unwanted side effects of currently available pharmaceutical drugs. This will be an important step towards truly personalized medicine.
Researchers: Jonas Frisén (KI), Jens Lagergren (KTH)
Background: To understand human development and pathological processes, one needs to understand how different cell types are generated and how they function. It is also vital to understand what drives these cell types to develop into specific phenotypes, whether it is determined by their lineage or by their surrounding microenvironment. LUSTRE, a technique developed by the Frisén lab, can track a specific marker segment (LINE-1) inside cells to reveal information about their lineage, which could help in the development of new pharmaceuticals targeting specific cells.
Aim: To track genomic alterations and reconstruct linage trees in human blood, brain, and cancer cells, by developing and optimizing phylogenetic methodologies that take advantage of modern deep-networks to sort out the underlying signal, from LUSTRE data, and to develop Variational Auto-Encoders (VAEs) to reduce experimental noise.
Methods: LUSTRE is used to track the LINE-1 elements in single cells, while gene expression is simultaneously analyzed with Smart-seq3, to explore lineage relationships in human tissue. Deep learning neural network-based methods are then applied to track genomic alterations at the single-cell level. Mitochondrial mutational profiles will also be used in combination with LUSTRE data to identify cell families and determine spatial localization.
Significance: The study holds significant implications for understanding human development and disease processes, and might reveal important information about different cell types, which can help develop new therapies targeting these specific cell types.
Researchers: Niklas Mattsson-Carlgren (LU), Kalle Åström (LU)
Background: Neurodegenerative diseases like Alzheimer’s and Parkinson’s are serious global health problems with no effective cures. Studying these diseases directly in patients is crucial, but the disease progression is slow, and the symptoms may vary from patient to patient. Developing biomarkers is one way to detect diseases early but due to legal and ethical concerns, sharing data is challenging. To address this problem, scientists are now exploring “synthetic cohorts”, which consists of virtual patient data sets. These virtual clinical-like data sets could be of vital importance to researchers with novel methodology but lacking patient data, help train AI models, enable “in silico” experiments, and for educational purposes.
Aim: To utilize machine learning models to create realistic “synthetic cohorts” of patients with neurodegenerative diseases, which will be shareable without violating current ethical and legal principles. The data from these models will also be analyzed to reveal new associations within neurodegenerative diseases, advancing our understanding of these conditions.
Methods: The generation of synthetic cohorts involves mathematical modeling using existing observational data. These models enable the creation of comprehensive, virtual patient datasets, allowing researchers worldwide to conduct experiments related to neurodegenerative diseases.
Significance: The development and global availability of “synthetic cohorts” play a pivotal role in advancing our understanding of neurodegenerative disease mechanisms. By identifying new associations and potential drug targets, these cohorts could accelerate therapy development. The knowledge gained from the project might also have a big impact on various other areas of medicine.
Researchers: Aleksej Zelezniak (Chalmers), Enric Llorens (KI)
Background: While gene and cell therapies show great potential in treating genetic disorders by targeting inherent genetic defects, effective regulation of therapeutic gene expression in a cell type-specific manner remains a challenge. Achieving precise control over the gene expression has the potential to significantly enhance both the safety and efficacy of those therapies, reducing side effects and health risks. One way to achieve this is to utilize advanced sequencing techniques and machine learning, particularly deep generative neural networks (DGNN), to design targeted regulatory DNA sequences, with the potential to revolutionize the field by enabling precise programmability of regulatory DNA.
Aim: To design and experimentally validate synthetic regulatory sequences for cell-specific gene expression control, using deep generative neural networks (DGNNs).
Methods: A comprehensive dataset for training the DGNNs will be developed by using a map of cell type-specific regulatory elements, such as enhancers, created by exploring single-cell chromatin accessibility data (ATAC-seq) and (RNA-seq). The regulatory sequences will be selected based on their specificity in controlling gene expression in therapeutically relevant cell types and validated through multiplexed single-cell enhancer reporter assays in stem cell-derived therapeutic cells.
Significance: If the project is successful, the AI-designed regulatory sequences might lead to greatly improved gene therapy by enabling precise control of gene expression in specific cell types.
Researchers: Nanna Holmgaard List (KTH), Talha Bin Masood (LiU)
Background: Sustaining almost all life on our planet and essential for visual perception, light plays a pivotal role in biology. At a molecular level, photo-responsive proteins are responsible for light-induced processes, whose biotechnological exploitations have already led to many life science breakthroughs. A deep understanding of these systems is crucial to understand photobiology and to engineer novel photoactive systems that can further advance life sciences.
Aim: The aim of the project is to combine automated quantum mechanics/molecular mechanics (QM/MM) workflows, topological data analysis, and visualization to investigate the workings of photoactive proteins. This three-pronged approach aims to decode complex data and understand not just “what happens” at the molecular level but also “why it happens.” The focus will be on proteins relevant to bioimaging and optogenetics.
Methods: The workflow for constructing reliable QM/MM models across sets of proteins will be optimized and improved significantly. The new models will also be used to create statistical and topological data analysis methods, capable of summarization, feature extraction, and robust comparison of time-evolving multifield data. An integrated visualization platform that combines analysis tools with innovative visual representations will also be developed.
Significance: The research will offer new insights into protein design factoring in photofunction with potential implications for a variety of technologies from biosensing to light-powered chemistry. Simultaneously, the project’s multifaceted analysis and visualization methods have broad applications, extending beyond biology to fields such as material and climate sciences.